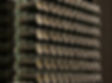
Introduction:
Light fields describe the amount of light flowing in every direction through every point in 3D space. In other words, this can be interpreted as many cameras taking photos of the same scene at different perspective views. Thus, light fields are technically a collection of photos/images taken at different angles. Light fields hold more information than a singular image, which is useful for many applications.
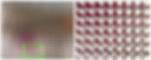
An example of a light field scene, composed of many different snapshots taken from different angles by a light field camera.
So what are these applications of light fields?
These applications all fall under the gigantic umbrella of computer science, and further falls under the subtopics of computer vision and machine learning. Some applications include synthetic aperture photography, 3D displays/reconstructions/models of objects such as holograms, and depth estimation.
Here are the definitions of each application:
Synthetic aperture photography or imaging basically involves projecting images from different views of a scene on a surface to reduce occlusions/obstructions in this scene.
Light field displays (LFDs) are created by using a high resolution panel or a projector array, and the result is a RGB-color video display that's in 3D.
Depth estimation is the process of calculating the depth of an object in an image.
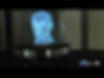
Picture of a 3D light field display from 360 degrees.
How are light fields related to machine learning?
Machine learning models, especially Convolutional Neural Networks, have been extensively used for image-based applications such as classification, identification, etc., but they can also be trained on light field scenes. For example, Shin et al. developed and used a CNN model, EPINET, to estimate the depth of light field images by training EPINET on the HCI 4D Light Field Benchmark dataset. ML for depth estimation has been seen to perceive depth faster and more accurately than other calculation-based methods. Furthermore, machine learning models can be very robust to noise (a lot of real-life light field data will have noise). Pei et al. also developed a deep neural network to estimate whether a single image is in focus or not for the task of synthetic aperture imaging.

Shin et al.’s EPINET architecture for depth estimation.
Conclusion As light field research advances, it could bring new inventions or enhance existing photographic and imaging tasks for not only scientists but also individuals as well. For example, we could have more enhanced image editing through image refocusing, we could see more 3D holograms created by light fields such as those from Marvel movies, etc.
Cited Sources (for images and text) -
front image- http://lightfield-forum.com/what-is-the-lightfield/
What is the light field? LightField Forum. (2019, January 18). Retrieved September 15, 2022, from http://lightfield-forum.com/what-is-the-lightfield/
Computer Graphics at Stanford University. (n.d.). Retrieved September 17, 2022, from https://graphics.stanford.edu/~vaibhav/pubs/thesis.pdf
Light field displays. Holoxica Limited. (n.d.). Retrieved September 17, 2022, from https://www.holoxica.com/light-field-displays
Shin, C., Jeon, H. G., Yoon, Y., Kweon, I. S., & Kim, S. J. (2018). Epinet: A fully-convolutional neural network using epipolar geometry for depth from light field images. In Proceedings of the IEEE Conference on Computer Vision and Pattern Recognition (pp. 4748–4757).
Z. Pei, L. Huang, Y. Zhang, M. Ma, Y. Peng and Y. -H. Yang, “Focus Measure for Synthetic Aperture Imaging Using a Deep Convolutional Network,” in IEEE Access, vol. 7, pp. 19762–19774, 2019, doi: 10.1109/ACCESS.2019.2896655.
Tonyee, & Tonyee. (n.d.). Adobe. ImageNation. Retrieved September 17, 2022, from https://tonyee.wordpress.com/tag/adobe/
YouTube. (2011). YouTube. Retrieved September 17, 2022, from https://www.youtube.com/watch?v=8gvPS1m40gw.
Zhou, S., Zhu, T., Shi, K., Li, Y., Zheng, W., & Yong, J. (2021). Review of light field technologies. Visual computing for industry, biomedicine, and art, 4(1), 29. https://doi.org/10.1186/s42492-021-00096-8